In today's ever-evolving technological landscape, security breaches are becoming increasingly prevalent. With Software-defined Networking (SDN) networks being a primary target for hackers, it's more important than ever to have strong security measures in place. Fortunately, the TeraFlow project has stepped up to the challenge by introducing enhanced security features for SDN networks through the use of Artificial Intelligence (AI) techniques.
TeraFlow leverages Deep Neural Network (DNN) models to analyze network traffic and detect attacks that might be occurring in the network in real-time. By doing so, it can swiftly identify and respond to potential security threats before they can cause any damage. With the use of AI, TeraFlow takes security measures to a whole new level and provides a robust shield against cyber attacks on software-defined networks.
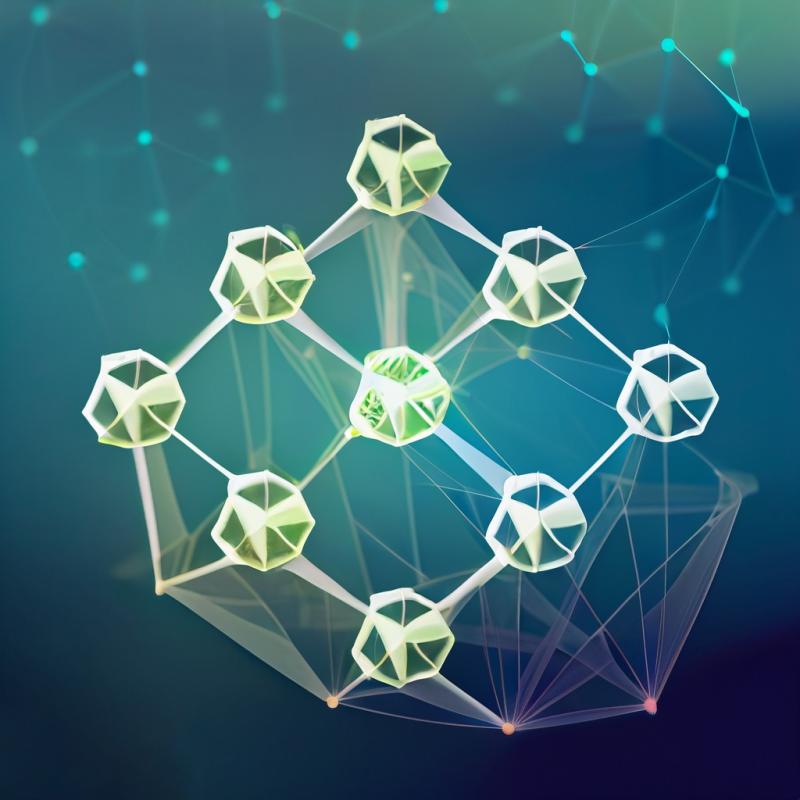
However, there's one major obstacle that comes with deploying DNN models in production networks: their high computational cost. This can lead to a significant amount of energy consumption over the model's lifetime, which in turn severely impacts the energy efficiency of the whole system. It's a concern that's especially important when dealing with real-time data processing, like in telecommunication systems. In these scenarios, buffering the data isn't always an option, which results in an increased number of inferences and, ultimately, more energy waste.
Reducing energy consumption and improving energy efficiency in the telecommunication industry is no longer an option - it's a necessity. With the industry's continued growth, it's crucial to manage energy sustainably to keep operating costs in check. Not only does the waste of energy resources negatively impact the environment, but it also affects the economy.
Driven by TeraFlow's commitment to reducing energy consumption and paving the way to a more sustainable future for SDN operations, significant progress has recently been made in optimizing the energy efficiency of DNN models integrated into the TeraFlow SDN controller.
One of the biggest challenges in optimizing DNNs is finding the right balance between accuracy, performance, and energy consumption. It is a delicate balancing act that requires the application and evaluation of various combinations of techniques. The TeraFlow project has been actively exploring different state-of-the art optimization techniques to find the most suitable set of methods that optimize this balance, including:
- Model pruning: This technique involves removing unnecessary or redundant parameters from the DNN, resulting in a smaller and more efficient model with minimal impact on accuracy.
- Quantization: This technique involves reducing the precision of the weights and activations in the DNN, resulting in a smaller model and lower energy consumption. However, this can also lead to a drop in accuracy.
- Knowledge distillation: This technique involves transferring knowledge from a larger and more accurate DNN (known as the teacher model) to a smaller and more efficient DNN (known as the student model). This can improve the performance and energy consumption of the student model without sacrificing accuracy.
- Neural Architecture search: This technique involves automatically searching for the best DNN architecture for a given task, considering trade-offs between accuracy, performance, and energy consumption.
These techniques have been extensively evaluated to ensure that they are effective in reducing the energy consumption of the DNN model responsible for detecting attacks in the network control and data planes while maintaining the expected accuracy and performance levels.
After conducting an extensive evaluation, we've been able to successfully identify the optimal set of optimization techniques that have helped us reduce the total energy consumption of the DNN-based attack detector by a staggering 82.304% during the inference phase, while maintaining a minimal loss of just 0.008% in our attack detection accuracy.
At TeraFlow, we are excited to continue our efforts towards improving sustainability and energy efficiency in the telecommunication industry, and we look forward to making more significant strides in this direction in the future. We hope that our efforts will inspire other companies and individuals in the telecommunication sector to join us in this journey towards sustainability. Together, we can create a better world for ourselves and future generations.